신용평가모형의 안정성 검증 지표의 하나인 PSI에 대한 자세한 설명은 2021.07.07 - [CSS(Credit Scoring System)/신용평가모형 검증지표] - [R실습]PSI 산출하기를 참고하세요. 본 포스팅에서는 파이썬을 활용해서 검증지표 산출만 실습해 보도록 하겠습니다.
1. 데이터 준비
실습을 위해 아래 자료를 다운로드 받으세요.
test_psi.csv
0.00MB
자료에 대한 설명은 상위 링크되어 있는 이전 포스팅을 참고하세요.
2. PSI 산출하기
import numpy as np
import pandas as pd
import math
# 실습 데이터 파이썬으로 불러오기
psi_test = pd.read_csv("D:/python_exer/test_psi.csv")
# 데이터 행 및 열의 개수 확인
psi_test .shape
# 대략적인 데이터 구조 확인
psi_test.head()
# 개발시점(2015.12) 등급별 건수 산출
grd_dist_dev = pd.DataFrame(psi_test[psi_test["kind"] == 201512].groupby("grade")["id"].count().reset_index())
# 검증 시점(2016.12) 등급별 건수 산출
grd_dist_dev = pd.DataFrame(psi_test[psi_test["kind"] == 201512].groupby("grade")["id"].count().reset_index())
# 'id' 열 이름을 'tot__cnt'로 변경
grd_dist_dev = grd_dist_dev.rename(columns = {"id" : "tot_cnt"})
grd_dist_val = grd_dist_val.rename(columns = {"id" : "tot_cnt"})
# 개발시점과 검증 시점의 등급별 구성비 산출
grd_dist_dev["tot_pct"] = grd_dist_dev["tot_cnt"] / grd_dist_dev["tot_cnt"].sum()
grd_dist_val["tot_pct"] = grd_dist_val["tot_cnt"] / grd_dist_val["tot_cnt"].sum()
# 개발 및 검증 시점 데이터를 신용등급 기준으로 결합하기
psi_table = pd.merge(grd_dist_dev, grd_dist_val, how="left", on="grade")
# 신용등급별 PSI 산출
for i in range(psi_table["grade"].size):
____psi_table.loc[i, "psi"] = (psi_table.loc[i,"tot_pct_x"] - psi_table.loc[i,"tot_pct_y"]) *
_____________________________math.log(psi_table.loc[i,"tot_pct_x"] / psi_table.loc[i,"tot_pct_y"])
# 최종 PSI 산출
round(psi_table.psi.sum(),8)
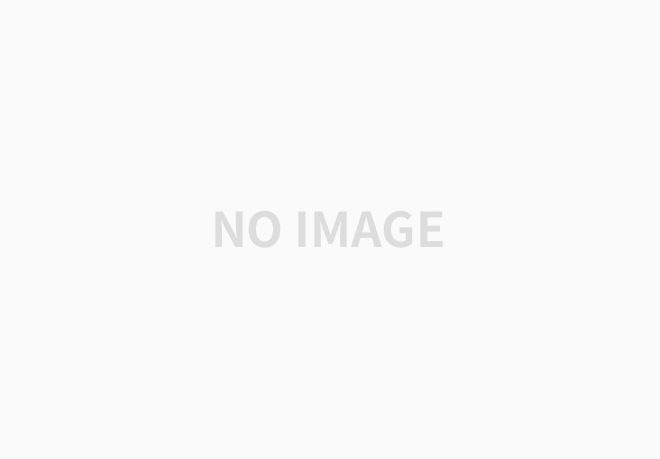
상위 링크에서 산출한 PSI 산출 값과 동일함을 알 수 있습니다.
반응형
'CSS(Credit Scoring System) > 신용평가모형 검증지표' 카테고리의 다른 글
[파이썬실습]신용평가 모형 검증(AUROC 산출하기) (0) | 2021.08.25 |
---|---|
[파이썬실습]신용평가 모형 검증(Information Value 산출하기) (0) | 2021.08.24 |
[파이썬실습]신용평가 모형 검증(K-S 통계량 산출하기) (0) | 2021.08.23 |
[R실습]PSI 산출하기 (0) | 2021.07.07 |
[R실습]AUROC 산출하기 (0) | 2021.07.04 |
댓글